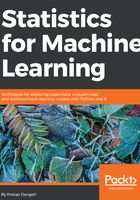
Machine learning models - ridge and lasso regression
In linear regression, only the residual sum of squares (RSS) is minimized, whereas in ridge and lasso regression, a penalty is applied (also known as shrinkage penalty) on coefficient values to regularize the coefficients with the tuning parameter λ.
When λ=0, the penalty has no impact, ridge/lasso produces the same result as linear regression, whereas λ -> ∞ will bring coefficients to zero:



Before we go deeper into ridge and lasso, it is worth understanding some concepts on Lagrangian multipliers. One can show the preceding objective function in the following format, where the objective is just RSS subjected to cost constraint (s) of budget. For every value of λ, there is an s such that will provide the equivalent equations, as shown for the overall objective function with a penalty factor:



Ridge regression works well in situations where the least squares estimates have high variance. Ridge regression has computational advantages over best subset selection, which requires 2P models. In contrast, for any fixed value of λ, ridge regression only fits a single model and the model-fitting procedure can be performed very quickly.
One disadvantage of ridge regression is it will include all the predictors and shrinks the weights according to their importance, but it does not set the values exactly to zero in order to eliminate unnecessary predictors from models; this issue is overcome in lasso regression. Given a situation where the number of predictors is significantly large, using ridge may provide accuracy, but it includes all the variables, which is not desired in a compact representation of the model; this issue is not present in lasso, as it will set the weights of unnecessary variables to zero.
Models generated from lasso are very much like subset selection, hence they are much easier to interpret than those produced by ridge regression.