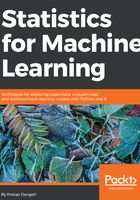
Backward and forward selection
There are various methods to add or remove variables to determine the best possible model.
In the backward method, iterations start with considering all the variables and we will remove variables one by one until all the prescribed statistics are met (such as no insignificance and multi-collinearity, and so on). Finally, the overall statistic will be checked, such as if R-squared value is > 0.7 , it is considered a good model, else reject it. In industry, practitioners mainly prefer to work on backward methods.
In the case of forward, we will start with no variables and keep on adding significant variables until the overall model's fit improves.
In the following method, we have used the backward selection method, starting with all the 11 independent variables and removing them one by one from analysis after each iteration (insignificant and multi-collinear variable):
>>> colnms = ['fixed_acidity', 'volatile_acidity', 'citric_acid', 'residual_sugar', 'chlorides', 'free_sulfur_dioxide', 'total_sulfur_dioxide', 'density', 'pH', 'sulphates', 'alcohol'] >>> pdx = wine_quality[colnms] >>> pdy = wine_quality["quality"]
Create the train and test data by randomly performing the data split. The random_state (random seed) is used for reproducible results:
>>> x_train,x_test,y_train,y_test = train_test_split(pdx, pdy, train_size = 0.7, random_state = 42)
In the following code, adding constant means creating an intercept variable. If we do not create an intercept, the coefficients will change accordingly:
>>> x_train_new = sm.add_constant(x_train) >>> x_test_new = sm.add_constant(x_test) >>> full_mod = sm.OLS(y_train,x_train_new)
The following code creates a model summary including R-squared, adjusted R-squared, and the p-value of independent variables:
>>> full_res = full_mod.fit() >>> print ("\n \n",full_res.summary())
The following code calculated VIF for all individual variables from first principles. Here we are calculating the R-squared value for each variable and converting it into a VIF value:
>>> print ("\nVariance Inflation Factor") >>> cnames = x_train.columns >>> for i in np.arange(0,len(cnames)): ... xvars = list(cnames) ... yvar = xvars.pop(i) ... mod = sm.OLS(x_train[yvar],sm.add_constant( x_train_new[xvars])) ... res = mod.fit() ... vif = 1/(1-res.rsquared) ... print (yvar,round(vif,3))
The preceding code generates the following output, from which we can start thinking about tuning the multilinear regression model.
Iteration 1:

The key metrics to focus on while tuning the model are AIC, adjusted R-squared, individual variable's P>|t|, and VIF values (shown as follows). Any model would be considered as good to go having the following thumb rule criteria:
- AIC: No absolute value is significant. It is a relative measure, the lower the better.
- Adjusted R-squared: It is ≥ 0.7.
- Individual variable's p-value (P>|t|): It is ≤ 0.05.
- Individual variable's VIF: It is ≤ 5 (in the banking industry, at some places, people use ≤ 2 as well).

By looking into the preceding results, residual_sugar has highest the p-value of 0.668 and fixed_acidity has the highest VIF value of 7.189. In this situation, always first remove the most insignificant variable, as insignificance is a more serious problem than multi-collinearity, though both should be removed while reaching the final model.
Run the preceding code after removing the residual_sugar variable from the columns list; we get the following result from iteration 2:
- AIC: Merely reduced from 2231 to 2229.
- Adjusted R-squared: Value did not change from 0.355.
- Individual variable's p-value (P>|t|): Density is still coming in as most insignificant with a value of 0.713.
- Individual variable's VIF: The fixed_acidity has the VIF ≥ 5. However, the density variable needs to be removed first, as priority is given to insignificance.
Iteration 2:


Based on iteration 2, we need to remove the density variable and rerun the exercise until no violation of p-value and VIF happens. We did skip the intermediate steps to save space; however, you are encouraged to manually do the step-by-step process of removing variables one by one.
The model could not be improved further after iteration 5, as it satisfies all the p-value and VIF criteria. The results are presented here.
Iteration 5:
In this example, we have got the final results after iteration 5:
- AIC: Reduced from 2231 from iteration 1 to 2225 in iteration 5.
- Adjusted R-squared: Value changed to 0.356, which is a slight improvement but not worth enough!
- Individual variable's p-value (P>|t|): None of the variables are insignificant; all values are less than 0.05.
- Individual variable's VIF: All variables are less than five. Hence, we do not need to remove any further variable based on VIF value.
We have got the answer that no strong relationship between the dependent and independent variables exists. However, we can still predict based on the testing data and calculate R-square to reconfirm.
The following code steps utilize the model to predict on testing data:
>>> # Prediction of data >>> y_pred = full_res.predict(x_test_new) >>> y_pred_df = pd.DataFrame(y_pred) >>> y_pred_df.columns = ['y_pred'] >>> pred_data = pd.DataFrame(y_pred_df['y_pred']) >>> y_test_new = pd.DataFrame(y_test) >>> y_test_new.reset_index(inplace=True) >>> pred_data['y_test'] = pd.DataFrame(y_test_new['quality'])
For R-square calculation, the scikit-learn package sklean.metrics module is utilized:
>>> # R-square calculation >>> rsqd = r2_score(y_test_new['quality'].tolist(), y_pred_df['y_pred'].tolist()) >>> print ("\nTest R-squared value:",round(rsqd,4))
The adjusted R-square value for test data appears as 0.3519, whereas the training R-square is 0.356. From the final results, we can conclude that the relationship between the independent variable and dependent variable (quality) is not able to be established with linear regression methodology.
The R code for linear regression on the wine data is as follows:
library(usdm) # Linear Regression wine_quality = read.csv("winequality-red.csv",header=TRUE,sep = ";",check.names = FALSE) names(wine_quality) <- gsub(" ", "_", names(wine_quality)) set.seed(123) numrow = nrow(wine_quality) trnind = sample(1:numrow,size = as.integer(0.7*numrow)) train_data = wine_quality[trnind,] test_data = wine_quality[-trnind,] xvars = c("volatile_acidity","chlorides","free_sulfur_dioxide", "total_sulfur_dioxide","pH","sulphates","alcohol") yvar = "quality" frmla = paste(yvar,"~",paste(xvars,collapse = "+")) lr_fit = lm(as.formula(frmla),data = train_data) print(summary(lr_fit)) #VIF calculation wine_v2 = train_data[,xvars] print(vif(wine_v2)) #Test prediction pred_y = predict(lr_fit,newdata = test_data) R2 <- 1 - (sum((test_data[,yvar]-pred_y )^2)/sum((test_data[,yvar]-mean(test_data[,yvar]))^2)) print(paste("Test Adjusted R-squared :",R2))