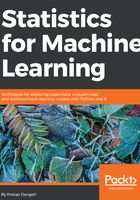
Example of simple linear regression using the wine quality data
In the wine quality data, the dependent variable (Y) is wine quality and the independent (X) variable we have chosen is alcohol content. We are testing here whether there is any significant relation between both, to check whether a change in alcohol percentage is the deciding factor in the quality of the wine:
>>> import pandas as pd >>> from sklearn.model_selection import train_test_split >>> from sklearn.metrics import r2_score >>> wine_quality = pd.read_csv("winequality-red.csv",sep=';') >>> wine_quality.rename(columns=lambda x: x.replace(" ", "_"), inplace=True)
In the following step, the data is split into train and test using the 70 percent - 30 percent rule:
>>> x_train,x_test,y_train,y_test = train_test_split (wine_quality ['alcohol'], wine_quality["quality"],train_size = 0.7,random_state=42)
After splitting a single variable out of the DataFrame, it becomes a pandas series, hence we need to convert it back into a pandas DataFrame again:
>>> x_train = pd.DataFrame(x_train);x_test = pd.DataFrame(x_test) >>> y_train = pd.DataFrame(y_train);y_test = pd.DataFrame(y_test)
The following function is for calculating the mean from the columns of the DataFrame. The mean was calculated for both alcohol (independent) and the quality (dependent) variables:
>>> def mean(values): ... return round(sum(values)/float(len(values)),2) >>> alcohol_mean = mean(x_train['alcohol']) >>> quality_mean = mean(y_train['quality'])
Variance and covariance is indeed needed for calculating the coefficients of the regression model:
>>> alcohol_variance = round(sum((x_train['alcohol'] - alcohol_mean)**2),2) >>> quality_variance = round(sum((y_train['quality'] - quality_mean)**2),2) >>> covariance = round(sum((x_train['alcohol'] - alcohol_mean) * (y_train['quality'] - quality_mean )),2) >>> b1 = covariance/alcohol_variance >>> b0 = quality_mean - b1*alcohol_mean >>> print ("\n\nIntercept (B0):",round(b0,4),"Co-efficient (B1):",round(b1,4))
After computing coefficients, it is necessary to predict the quality variable, which will test the quality of fit using R-squared value:
>>> y_test["y_pred"] = pd.DataFrame(b0+b1*x_test['alcohol']) >>> R_sqrd = 1- ( sum((y_test['quality']-y_test['y_pred'])**2) / sum((y_test['quality'] - mean(y_test['quality']))**2 )) >>> print ("Test R-squared value",round(R_sqrd,4))

From the test R-squared value, we can conclude that there is no strong relationship between quality and alcohol variables in the wine data, as R-squared is less than 0.7.
Simple regression fit using first principles is described in the following R code:
wine_quality = read.csv("winequality-red.csv",header=TRUE,sep = ";",check.names = FALSE) names(wine_quality) <- gsub(" ", "_", names(wine_quality)) set.seed(123) numrow = nrow(wine_quality) trnind = sample(1:numrow,size = as.integer(0.7*numrow)) train_data = wine_quality[trnind,] test_data = wine_quality[-trnind,] x_train = train_data$alcohol;y_train = train_data$quality x_test = test_data$alcohol; y_test = test_data$quality x_mean = mean(x_train); y_mean = mean(y_train) x_var = sum((x_train - x_mean)**2) ; y_var = sum((y_train-y_mean)**2) covariance = sum((x_train-x_mean)*(y_train-y_mean)) b1 = covariance/x_var b0 = y_mean - b1*x_mean pred_y = b0+b1*x_test R2 <- 1 - (sum((y_test-pred_y )^2)/sum((y_test-mean(y_test))^2)) print(paste("Test Adjusted R-squared :",round(R2,4)))