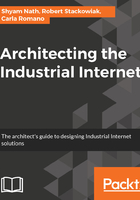
Data and analytics
IIoT solutions rely heavily on the use of advanced analytics on the data collected from the operational systems. This data is often merged with the data from the enterprise systems. Typically, the volume of data from the operation systems may far exceed the data from the latter source. Let's take an example of data from aircraft. According to Aviation Week, a modern generation aircraft can generate over one terabyte of data in a cross-country flight. Modern generation aircraft can have more than 1,000 sensors, including about 300 in the jet engine alone (http://aviationweek.com/connected-aerospace/internet-aircraft-things-industry-set-be-transformed).
This poses several kinds of challenges for the data and analytics design in the case of IIoT systems. Here are a few considerations:
- Storage of data: The sheer volume of data that can be generated by recording all the sensor readings at the rate of even 1/16 of a second makes it hard to store all the data on board an aircraft, for a single flight.
- Transfer of data: Due to the very high cost of secured communication channels between the aircraft and ground systems, it is not feasible to transfer full data with wireless, while the aircraft is in flight. Thus, only summary data in the range of a few kilobytes is transferred via the wireless medium. The bulk of the data collected on board the aircraft is transferred once the aircraft lands.
- Data semantics: The data that is collected from the aircraft at the airport must be decrypted and assigned meaning. In addition, engineering units must be added to this data before it is useful for any meaningful analysis.
- Data volume: Once the data from the single aircraft is collected for one flight, it must be sent to a big data store to allow its use with prior data for the same aircraft or engine. Such big data systems can quickly reach to petabytes or more, as data from the whole fleet of aircraft is collected, even say for 90 days.
- Challenges for analytics: Due to the nature of sensor data and its sheer volume, the data scientists face several challenges in coming up with meaningful analytics. They cannot pull large amounts of data where the analytics process runs in the server's memory and needs to load all the data for analysis. An example would be a Java program trying to cycle through terabytes of data. Instead, the computing paradigm shifts to near-data analytics as shown in Figure 2.13. On the right-hand side, we can see that big data systems such as Hadoop allow the analytics process to run near data, alleviating the need to pull large amounts of data into memory:

- Merging OT and IT data: We discussed how to handle a large amount of operational or sensor data using the near-data analytics paradigm. However, the IT systems or enterprise data, for example, who owns this aircraft or the prior history of this engine, will often reside in relational systems. It is not easy to merge OT and the IT data in a meaningful way. To achieve the full potential of IIoT systems, it is important to bring all the relevant information or the enterprise context of the data in one place before it is fed to the analytics engines.
- Multiplicity of Analytics languages: Finally, most mature organizations have a proliferation of languages and tools for advanced analytics. Commonly used languages and environments may include, C/C++, Java, Matlab, Python, R, and so on. Thus, the analytics platform for IIoT systems should ideally be able to deploy analytics and analytical workflows that are written in these common languages.