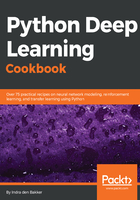
上QQ阅读APP看书,第一时间看更新
How to do it...
- At the moment, gluon is included in the latest release of MXNet (follow the steps in Building efficient models with MXNet to install MXNet).
- After installing, we can directly import gluon as follows:
from mxnet import gluon
- Next, we create some dummy data. For this we need the data to be in MXNet's NDArray or Symbol:
import mxnet as mx
import numpy as np
x_input = mx.nd.empty((1, 5), mx.gpu())
x_input[:] = np.array([[1,2,3,4,5]], np.float32)
y_input = mx.nd.empty((1, 5), mx.gpu())
y_input[:] = np.array([[10, 15, 20, 22.5, 25]], np.float32)
- With Gluon, it's really straightforward to build a neural network by stacking layers:
net = gluon.nn.Sequential()
with net.name_scope():
net.add(gluon.nn.Dense(16, activation="relu"))
net.add(gluon.nn.Dense(len(y_input)))
- Next, we initialize the parameters and we store these on our GPU as follows:
net.collect_params().initialize(mx.init.Normal(), ctx=mx.gpu())
- With the following code we set the loss function and the optimizer:
softmax_cross_entropy = gluon.loss.SoftmaxCrossEntropyLoss()
trainer = gluon.Trainer(net.collect_params(), 'adam', {'learning_rate': .1})
- We're ready to start training or model:
n_epochs = 10
for e in range(n_epochs):
for i in range(len(x_input)):
input = x_input[i]
target = y_input[i]
with mx.autograd.record():
output = net(input)
loss = softmax_cross_entropy(output, target)
loss.backward()
trainer.step(input.shape[0])
We've shortly demonstrated how to implement a neural network architecture with Gluon. Gluon is a powerful extension that can be used to implement deep learning architectures with clean code. At the same time, there is almost no performance loss when using Gluon.