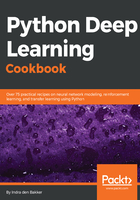
上QQ阅读APP看书,第一时间看更新
How to do it...
- First, we will show how to install TensorFlow from your terminal (make sure that you adjust the link to the TensorFlow wheel for your platform and Python version accordingly):
pip install --ignore-installed --upgrade https://storage.googleapis.com/tensorflow/linux/gpu/tensorflow_gpu-1.3.0-cp35-cp35m-linux_x86_64.whl
This will install the GPU-enabled version of TensorFlow and the correct dependencies.
- You can now import the TensorFlow library into your Python environment:
import tensorflow as tf
- To provide a dummy dataset, we will use numpy and the following code:
import numpy as np
x_input = np.array([[1,2,3,4,5]])
y_input = np.array([[10]])
- When defining a TensorFlow model, you cannot feed the data directly to your model. You should create a placeholder that acts like an entry point for your data feed:
x = tf.placeholder(tf.float32, [None, 5])
y = tf.placeholder(tf.float32, [None, 1])
- Afterwards, you apply some operations to the placeholder with some variables. For example:
W = tf.Variable(tf.zeros([5, 1]))
b = tf.Variable(tf.zeros([1]))
y_pred = tf.matmul(x, W)+b
- Next, define a loss function as follows:
loss = tf.reduce_sum(tf.pow((y-y_pred), 2))
- We need to specify the optimizer and the variable that we want to minimize:
train = tf.train.GradientDescentOptimizer(0.0001).minimize(loss)
- In TensorFlow, it's important that you initialize all variables. Therefore, we create a variable called init:
init = tf.global_variables_initializer()
We should note that this command doesn't initialize the variables yet; this is done when we run a session.
- Next, we create a session and run the training for 10 epochs:
sess = tf.Session()
sess.run(init)
for i in range(10):
feed_dict = {x: x_input, y: y_input}
sess.run(train, feed_dict=feed_dict)
- If we also want to extract the costs, we can do so by adding it as follows:
sess = tf.Session()
sess.run(init)
for i in range(10):
feed_dict = {x: x_input, y: y_input}
_, loss_value = sess.run([train, loss], feed_dict=feed_dict)
print(loss_value)
- If we want to use multiple GPUs, we should specify this explicitly. For example, take this part of code from the TensorFlow documentation:
c = []
for d in ['/gpu:0', '/gpu:1']:
with tf.device(d):
a = tf.constant([1.0, 2.0, 3.0, 4.0, 5.0, 6.0], shape=[2, 3])
b = tf.constant([1.0, 2.0, 3.0, 4.0, 5.0, 6.0], shape=[3, 2])
c.append(tf.matmul(a, b))
with tf.device('/cpu:0'):
sum = tf.add_n(c)
# Creates a session with log_device_placement set to True.
sess = tf.Session(config=tf.ConfigProto(log_device_placement=True))
# Runs the op.
print(sess.run(sum))
As you can see, this gives a lot of flexibility in how the computations are handled and by which device.
This is just a brief introduction to how TensorFlow works. The granular level of model implementation gives the user a lot of flexibility when implementing networks. However, if you're new to neural networks, it might be overwhelming. That is why the Keras framework--a wrapper on top of TensorFlow—can be a good alternative for those who want to start building neural networks without getting too much into the details. T herefore, i n this book, the first few chapters will mainly focus on Keras, while the more advanced chapters will include more recipes that use other frameworks such as TensorFlow.