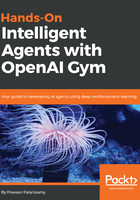
What is OpenAI Gym?
OpenAI Gym is an open source toolkit that provides a diverse collection of tasks, called environments, with a common interface for developing and testing your intelligent agent algorithms. The toolkit introduces a standard Application Programming Interface (API) for interfacing with environments designed for reinforcement learning. Each environment has a version attached to it, which ensures meaningful comparisons and reproducible results with the evolving algorithms and the environments themselves.
The Gym toolkit, through its various environments, provides an episodic setting for reinforcement learning, where an agent's experience is broken down into a series of episodes. In each episode, the initial state of the agent is randomly sampled from a distribution, and the interaction between the agent and the environment proceeds until the environment reaches a terminal state. Do not worry if you are not familiar with reinforcement learning. You will be introduced to reinforcement learning in Chapter 2, Reinforcement Learning and Deep Reinforcement Learning.
Some of the basic environments available in the OpenAI Gym library are shown in the following screenshot:

At the time of writing this book, the OpenAI Gym natively has about 797 environments spread over different categories of tasks. The famous Atari category has the largest share with about 116 (half with screen inputs and half with RAM inputs) environments! The categories of tasks/environments supported by the toolkit are listed here:
- Algorithmic
- Atari
- Board games
- Box2D
- Classic control
- Doom (unofficial)
- Minecraft (unofficial)
- MuJoCo
- Soccer
- Toy text
- Robotics (newly added)
The various types of environment (or tasks) available under the different categories, along with a brief description of each environment, is given next. Keep in mind that you may need some additional tools and packages installed on your system to run environments in each of these categories. Do not worry! We will go over every single step you need to do to get any environment up and running in the upcoming chapters. Stay tuned!
We will now see the previously mentioned categories in detail, as follows:
- Algorithmic environments: They provide tasks that require an agent to perform computations, such as the addition of multi-digit numbers, copying data from an input sequence, reversing sequences, and so on.
- Atari environments: These offer interfaces to several classic Atari console games. These environment interfaces are wrappers on top of the Arcade Learning Environment (ALE). They provide the game's screen images or RAM as input to train your agents.
- Board games: This category has the environment for the popular game Go on 9 x 9 and 19 x 19 boards. For those of you who have been following the recent breakthroughs by Google's DeepMind in the game of Go, this might be very interesting. DeepMind developed an agent named AlphaGo, which used reinforcement learning and other learning and planning techniques, including Monte Carlo tree search, to beat the top-ranked human Go players in the world, including Fan Hui and Lee Sedol. DeepMind also published their work on AlphaGo Zero, which was trained from scratch, unlike the original AlphaGo, which used sample games played by humans. AlphaGo Zero surpassed the original AlphaGo's performance. Later, AlphaZero was published; it is an autonomous system that learned to play chess, Go, and Shogi using self-play (without any human supervision for training) and reached performance levels higher than the previous systems developed.
- Box2D: This is an open source physics engine used for simulating rigid bodies in 2D. The Gym toolkit has a few continuous control tasks that are developed using the Box2D simulator:

The tasks include training a bipedal robot to walk, navigating a lunar lander to its landing pad, and training a race car to drive around a race track. Exciting! In this book, we will train an AI agent using reinforcement learning to drive a race car around the track autonomously! Stay tuned.
- Classic control: This category has many tasks developed for it and was used widely in reinforcement learning literature in the past. These tasks formed the basis for some of the early development and benchmarking of reinforcement learning algorithms. For example, one of the environments available under the classic control category is the Mountain Car environment, which was first introduced in 1990 by Andrew Moore (Dean of the School of Computer Science at CMU, and Pittsburgh founder) in his PhD thesis. This environment is still used sometimes as a test bed for reinforcement learning algorithms. You will create your first OpenAI Gym environment from this category in just a few moments towards the end of this chapter!
- Doom: This category provides an environment interface for the popular first-person shooter game Doom. It is an unofficial, community-created Gym environment category and is based on ViZDoom, which is a Doom-based AI research platform providing an easy-to-use API suitable for developing intelligent agents from raw visual inputs. It enables the development of AI bots that can play several challenging rounds of the Doom game using only the screen buffer! If you have played this game, you know how thrilling and difficult it is to progress through some of the rounds without losing lives in the game! Although this is not a game with cool graphics like some of the new first-person shooter games, the visuals aside, it is a great game. In recent times, several studies in machine learning, especially in deep reinforcement learning, have utilized the ViZDoom platform and have developed new algorithms to tackle the goal-directed navigation problems encountered in the game. You can visit ViZDoom's research web page (http://vizdoom.cs.put.edu.pl/research) for a list of research studies that use this platform. The following screenshot lists some of the missions that are available as separate environments in the Gym for training your agents:

- MineCraft: This is another great platform. Game AI developers especially might be very much interested in this environment. MineCraft is a popular video game among hobbyists. The MineCraft Gym environment was built using Microsoft's Malmo project, which is a platform for artificial intelligence experimentation and research built on top of Minecraft. Some of the missions that are available as environments in the OpenAI Gym are shown in the following screenshot. These environments provide inspiration for developing solutions to challenging new problems presented by this unique environment:

- MuJoCo: Are you interested in robotics? Do you dream of developing algorithms that can make a humanoid walk and run, or do a backflip like Boston Dynamic's Atlas Robot? You can! You will be able to apply the reinforcement learning methods you will learn in this book in the OpenAI Gym MuJoCo environment to develop your own algorithm that can make a 2D robot walk, run, swim, or hop, or make a 3D multi-legged robot walk or run! In the following screenshot, there are some cool, real-world, robot-like environments available under the MuJoCo environment:

- Soccer: This an environment suitable for training multiple agents that can cooperate together. The soccer environments available through the Gym toolkit have continuous state and action spaces. Wondering what that means? You will learn all about it when we talk about reinforcement learning in the next chapter. For now, here is a simple explanation: a continuous state and action space means that the action that an agent can take and the input that the agent receives are both continuous values. This means that they can take any real number value between, say, 0 and 1 (0.5, 0.005, and so on), rather than being limited to a few discrete sets of values, such as {1, 2, 3}. There are three types of environment. The plain soccer environment initializes a single opponent on the field and gives a reward of +1 for scoring a goal and 0 otherwise. In order for an agent to score a goal, it will need to learn to identify the ball, approach the ball, and kick the ball towards the goal. Sound simple enough? But it is really hard for a computer to figure that out on its own, especially when all you say is +1 when it scores a goal and 0 in any other case. It does not have any other clues! You can develop agents that will learn all about soccer by themselves and learn to score goals using the methods that you will learn in this book.
- Toy text: OpenAI Gym also has some simple text-based environments under this category. These include some classic problems such as Frozen Lake, where the goal is to find a safe path to cross a grid of ice and water tiles. It is categorized under toy text because it uses a simpler environment representation—mostly through text.
With that, you have a very good overview of all the different categories and types of environment that are available as part of the OpenAI Gym toolkit. It is worth noting that the release of the OpenAI Gym toolkit was accompanied by an OpenAI Gym website (gym.openai.com), which maintained a scoreboard for every algorithm that was submitted for evaluation. It showcased the performance of user-submitted algorithms, and some submissions were also accompanied by detailed explanations and source code. Unfortunately, OpenAI decided to withdraw support for the evaluation website. The service went offline in September 2017.
Now you have a good picture of the various categories of environment available in OpenAI Gym and what each category provides you with. Next, we will look at the key features of OpenAI Gym that make it an indispensable component in many of today's advancements in intelligent agent development, especially those that use reinforcement learning or deep reinforcement learning.