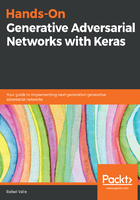
Deep learning basics
Deep learning is a subset of machine learning, which is a field of artificial intelligence that uses mathematics and computers to learn from data and map it from some input to some output. Loosely speaking, a map or a model is a function with parameters that maps the input to an output. Learning the map, also known as mode, occurs by updating the parameters of the map such that some expected empirical loss is minimized. The empirical loss is a measure of distance between the values predicted by the model and the target values given the empirical data.
Notice that this learning setup is extremely powerful because it does not require having an explicit understanding of the rules that define the map. An interesting aspect of this setup is that it does not guarantee that you will learn the exact map that maps the input to the output, but some other maps, as expected, predict the correct output.
This learning setup, however, does not come without a price: some deep learning methods require large amounts of data, specially when compared with methods that rely on feature engineering. Fortunately, there is a large availability of free data, specially unlabeled, in many domains.
Meanwhile, the term deep learning refers to the use of multiple layers in an ANN to form a deep chain of functions. The term ANN suggests that such models informally draw inspiration from theoretical models of how learning could happen in the brain. ANNs, also referred to as deep neural networks, are the main class of models considered in this book.