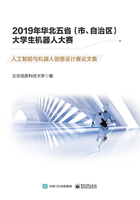
语音信号分解与混沌建模的识别技术研究
杨淑莹1, 2,李军广1, 2,邓东升1, 2,桂彬彬1, 2
1. 天津理工大学计算机科学与工程学院,天津市,300384;
2. 计算机视觉与系统教育部重点实验室,天津市,300384
摘要:语音生成过程属于非线性系统,语音信号具有混沌特性。传统的Volterra模型一般是二阶截断的,低阶滤波器用于估计语音信号,其预测效果不准确。因此,本文提出了一种基于小波包分解和Volterra自适应模型的特征提取方案。首先,语音信号将被小波包分解;其次,重建所有子带信号的相空间;最后,利用二阶Volterra级数展开和线性自适应FIR滤波器估计Volterra模型的参数向量H(n)和输出信号U(n),得到Volterra滤波器的权向量用于语音识别,基于隐马尔可夫模型完成语音识别实验。实验结果表明,提取的特征得到明显改善,特别是在噪声环境中。
关键词:小波包分解;相空间;Volterra自适应模型;语音识别;FIR滤波;隐马尔可夫模型;最小二乘;LPCC
Research on recognition technology of speech signaldecomposition and chaos modeling
Yang Shuying1, 2,Li Junguang1, 2,Deng Dongsheng1, 2,Gui Binbin1, 2
1. Tianjin University of Technology, School of Computer Science&Engineering, Tianjin City, 300384;
2.Key Laboratory of Computer Vision&System, Ministry of Education, Tianjin City, 300384
Abstract:The process of voice generation belongs to nonlinear system, and the voice signal is chaotic. The traditional Volterra model is generally 2-order truncation, a low order filter is used to estimate the speech signal, and the prediction effect is not accurate. So, this paper proposes a feature extraction scheme based on the wavelet packet decomposition and Volterra adaptive model. First, the speech signal will be decomposed by wavelet packet. Second, reconstruct the phase space of all sub-band signals. Third, using second order Volterra series expansion and the linear adaptive FIR filter to estimate parameter vector H(n)and output signal U(n)for Volterra model, and weight vectors of Volterra filter are obtained for speaker recognition. Speaker recognition experiment is completed based on hidden Markov model. The experimental results show that the extracted features have obviously improved, especially in the noise environment.
Keywords:Wavelet packet decomposition; Phase space; Volterra adaptive model; Speech recognition;FIR filtering;Hidden Markov model;Least squares;LPCC